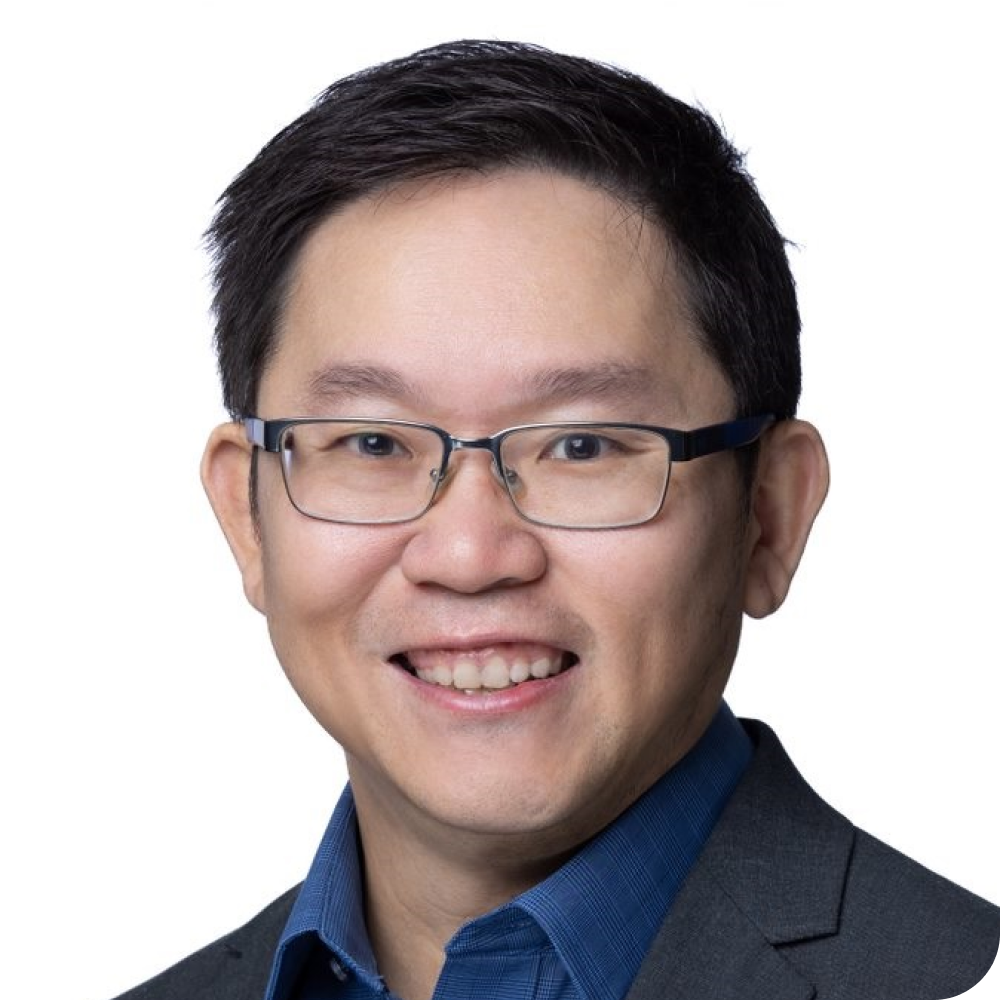
Distinguished Speaker Seminar
Speaker: Dr Tok Wee Hyong, Partner Director of Products with the Cloud and AI organization at Microsoft
Date: Wednesday November 6, 2024
Time: 2:30 PM – 4:00 PM SGT
Venue: COM3 Multi-purpose Hall #01-27
Abstract:
The invention of the Transformer architecture in the seminal paper “Attention is All You Need” has catalyzed the exciting momentum we are seeing today in Generative AI. Generative AI has enabled many innovative applications, which are fundamentally changing the way we learn, work and play. This creates opportunities for everyone, and for every industry. A key ingredient to training large (and small) language models is data. Data fuels AI, and it is important for every organization to have a holistic Data and AI strategy, building on a strong data foundation, that will meet every organization’s AI needs.
Using practical examples, you will get up to speed on the “unreasonable effectiveness” of Data and Generative AI. This talk brings you on an exciting journey on the past, present, and future of Generative AI, sharing best practices on translating Data and AI strategy to concrete outcomes that matter. Most importantly, you will learn how we can all get started today!
Biography:
Dr. Tok Wee Hyong is Partner Director of Products, with the Cloud and AI organisation at Microsoft. He is a seasoned Data and AI leader with a proven track record of running successful businesses and growing it from zero to sustained industry leadership positions. He is the author of more than 10+ books, covering topics ranging from products and artificial intelligence including: “Practical Weak Supervision”, Practical Automated Machine Learning”, and more. Prior to his current role, Wee Hyong led AI strategy and innovation. He was Head of AI Labs, where he led a global team of data scientists to deliver cutting-edge AI solutions from customers spanning different industries. Wee Hyong co-founded the AI for Earth Engineering and Data Science team, which seeded the foundation for many of the AI for Good initiatives, in using AI to solve some of the world’s toughest sustainability challenges using AI/ML.
Wee Hyong has a PhD in Computer Science from National University of Singapore.